Building Multilingual ML Models
Best Translation Services
03
Building Multilingual ML Models: Best Translation Services
Hello there! Imagine embarking on a journey to build multilingual machine learning (ML) models. It’s like navigating a vast ocean with countless opportunities and a few challenges along the way. But don’t worry, we’re here to guide you through every step. In this article, we’ll explore the best translation services and techniques to create powerful multilingual ML models. Ready to dive in? Let’s set sail!
The Rising Tide of Multilingual ML Models
Artificial Intelligence (AI) is a transformative field in computer science. It focuses on solving cognitive problems related to human intelligence, such as learning, creativity, and image recognition. Since its inception in the mid-20th century, AI technology has significantly evolved, offering numerous advantages like solving complex problems, enhancing business efficiency, making smarter decisions, and automating processes. Among these advancements, multilingual ML models have become increasingly significant.
Why Multilingual ML Models?
As the world becomes more interconnected, businesses and organizations need to communicate effectively across language barriers. Multilingual ML models enable applications to understand and generate content in multiple languages, opening doors to global markets and audiences. They play a crucial role in various industries, ensuring that language is no longer a barrier to innovation and efficiency.
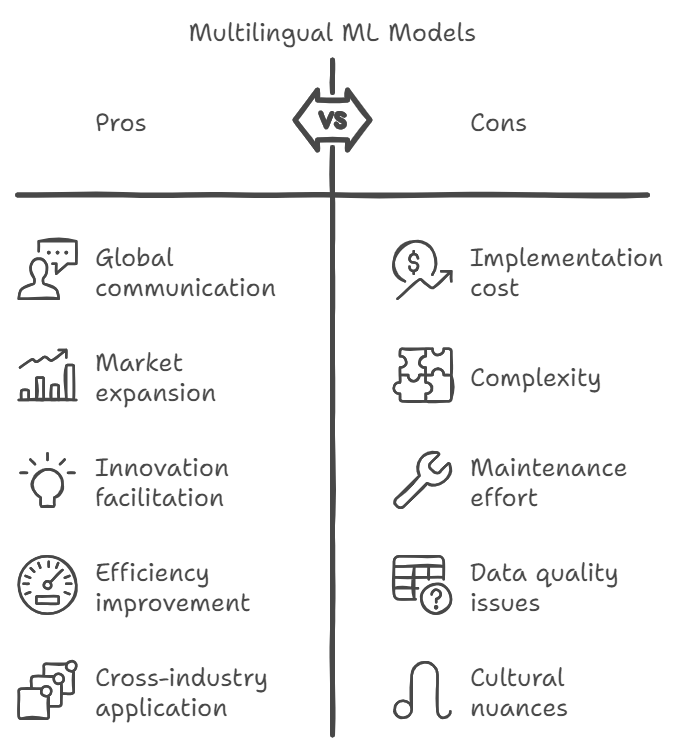
Key Technologies in Multilingual AI
To build robust multilingual ML models, several key AI technologies come into play:
- Deep Learning Neural Networks: These networks mimic the human brain’s structure and function, allowing models to learn from vast amounts of data.
- Natural Language Processing (NLP): This technology enables machines to understand and generate human language, making it essential for translation services.
- Computer Vision: While primarily used for image recognition, computer vision can also assist in translating text within images.
- Generative AI: This technology generates new content based on existing data, useful for creating translations that sound natural.
- Voice Recognition: Converts spoken language into text, facilitating real-time translation services.
Practical Applications Across Industries
Multilingual ML models have a wide range of applications:
- Healthcare: Assisting in patient communication and translating medical records.
- Finance: Enabling multilingual customer support and translating financial documents.
- Retail: Enhancing customer experience by providing product descriptions in multiple languages.
- Construction: Improving job site safety by translating safety guidelines and manuals.
Overcoming Challenges in Multilingual AI
While the benefits are immense, the path to building multilingual ML models is not without challenges. Key issues include:
- Data Governance: Ensuring data privacy and compliance with regulations.
- Technical Difficulties: Handling the complexities of different languages and scripts.
- Data Limitations: Accessing high-quality, diverse training data.
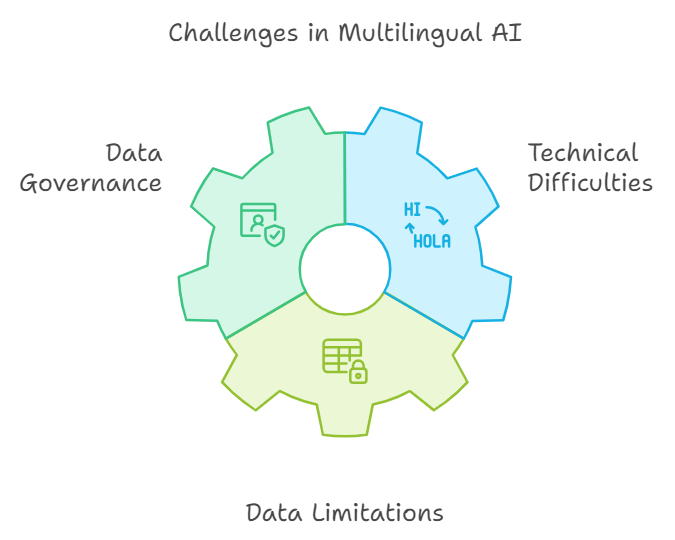
The Importance of High-Quality Data
The development of AI systems heavily relies on high-quality, diverse training data. Let’s break down what makes data high-quality:
- Accuracy: Data must be correct and free of errors.
- Consistency: Data should be uniform across different sources.
- Completeness: All necessary data points should be included.
- Timeliness: Data should be up-to-date.
- Uniqueness: Each data point should be distinct to avoid redundancy.
Ensuring data quality minimizes biases and errors, prevents overfitting, and ensures reliable outcomes. Diversity in data allows AI models to learn from a wide range of data points, improving accuracy and fairness.
Techniques to Enhance Data Diversity
Here are some techniques to enhance data diversity:
- Data Augmentation: Modifying existing data to create new training examples.
- Synthetic Data Generation: Using GANs (Generative Adversarial Networks) and VAEs (Variational Autoencoders) to create synthetic data.
- Collaboration with Partners: Partnering with other organizations to access diverse datasets.
The Role of Synthetic Data
Synthetic data, which mimics real-world data without containing actual information, offers several advantages:
- Unlimited Data Generation: Create as much data as needed for training.
- Privacy Protection: No real personal information is used, ensuring privacy.
- Bias Reduction: Synthetic data can be designed to reduce biases present in real-world data.
AWS supports synthetic data generation through services like Amazon SageMaker, which provides tools for data labeling and creating high-quality datasets.
Addressing Bias in AI Models
AI models must address inherent biases in training data, which can lead to unfair and unethical outcomes. Techniques to mitigate bias include:
- Ensuring Diverse Training Datasets: Including a wide range of data points.
- Regular Auditing: Continuously monitoring and reviewing models.
- Implementing Governance Frameworks: Establishing guidelines and policies for ethical AI use.
Organizations like DARPA and initiatives like the AI Next campaign are exploring new AI theories and applications to enhance contextual reasoning and adaptability in AI systems.
The Role of Leading Companies
High-quality AI training data is crucial for developing accurate and fair models. Companies like Appen and Shaip specialize in providing diverse and ethically sourced data for various AI applications, ensuring data privacy and compliance with standards like ISO and HIPAA. Oracle Cloud Infrastructure offers scalable resources to support complex AI model training, emphasizing the importance of explainability and human oversight in AI development.
A Growing Concern: Data Quality Standards
As AI’s role in various sectors, such as healthcare, construction, and finance, expands, there is a growing concern about the potential depletion of high-quality data for training AI models. Researchers suggest redefining data quality standards and incorporating diverse datasets to address this issue.
Latest Words
In summary, the successful implementation of AI hinges on high-quality, diverse training data, robust governance frameworks, and continuous innovation. Organizations must balance the benefits of AI with ethical considerations to ensure fair and responsible use of this transformative technology. Now that you’ve journeyed through the world of multilingual ML models, it’s time to test your knowledge!
Quiz Time!
- What are some key AI technologies used in building multilingual ML models?
- Name at least two industries where multilingual ML models can be applied.
- What are some challenges faced in implementing AI?
- How can synthetic data help in AI training?
- Why is data diversity important in training AI models?
Share your answers in the comments below!