The Evolution of Machine Translation
From Turing to Today
18
Hey there! Imagine the world where you can seamlessly communicate with anyone, regardless of language barriers. This dream is becoming a reality thanks to the fascinating journey of Machine Translation (MT). Let’s dive into this story, exploring how MT has evolved over the years, its applications, and what the future holds for this incredible technology.
The Birth of AI and Early Machine Translation
Our story begins in the 1950s with Alan Turing, often dubbed the father of artificial intelligence (AI). Turing’s groundbreaking work laid the foundation for what we now know as AI. Initially, AI aimed to solve cognitive problems similar to human intelligence, such as learning, creativity, and image recognition. This was the era of rule-based systems in MT, where translations followed predefined rules and dictionaries.
Key Milestones in Early MT:
- 1950s: Alan Turing’s foundational work.
- 1960s-70s: Development of rule-based MT systems.
- 1980s: Introduction of statistical machine translation (SMT).
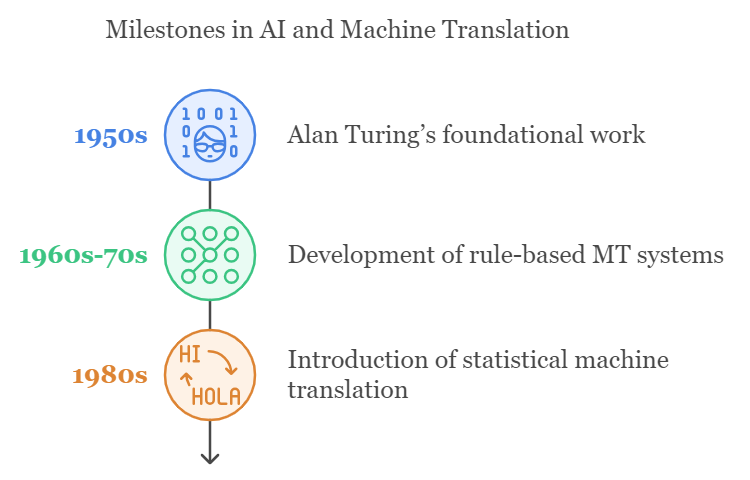
Modern AI and the Rise of Neural Machine Translation (NMT)
Fast forward to today, AI has grown leaps and bounds, encompassing technologies like deep neural networks, natural language processing (NLP), and computer vision. One of the most transformative advancements in MT is Neural Machine Translation (NMT). NMT utilizes deep learning to provide more accurate and contextually appropriate translations compared to its predecessors.
Major Platforms Leveraging NMT
- Google Translate: Offers fast, high-quality translations across numerous languages.
- DeepL: Known for its nuanced and contextually accurate translations.
- Amazon Translate: Integrates seamlessly with various applications for real-time translation.
Despite these advancements, capturing cultural nuances and contextual accuracy remains a challenge, often requiring human intervention for quality assurance.
Applications and Impact
The impact of machine translation and AI-driven language services is nothing short of revolutionary. These technologies have transformed multilingual communication, enabling real-time translation and supporting global business operations.
Enhancing Efficiency and Accuracy
AI tools assist in various aspects of translation, making the process more efficient and accurate. Platforms like Smartcat and Phrase provide collaborative environments for translation tasks, integrating AI to streamline workflows and boost productivity.
How AI Improves Translation:
- Terminology Management: Ensures consistent use of terms.
- Error Detection: Identifies and corrects mistakes.
- Quality Control: Maintains high standards of translation accuracy.
Industry-Specific Innovations
AI and MT technologies are making waves across different sectors, each benefiting uniquely from these advancements.
Healthcare
AI is revolutionizing healthcare by aiding in diagnostics, predictive analytics, and personalized medicine. For instance, Amazon HealthLake helps analyze health data to improve patient outcomes.
Finance
In the finance sector, AI enhances fraud detection, risk assessment, and customer service through chatbots and automated processes.
Retail
Retailers are leveraging AI-driven inventory management to optimize stock levels and supply chain operations, while personalized recommendations enhance customer experience.
Agriculture
AI technologies, like those from Labby Inc., are used for real-time milk quality testing, helping farmers detect issues early and improve productivity.
Ensuring Quality Control and Evaluation
High-quality translation and AI outputs are crucial for effective communication. Several strategies are employed to ensure this quality:
- Simplifying Input Text: Reduces ambiguity and improves translation accuracy.
- Training AI Models: Using domain-specific data to enhance performance.
- Translation Memories: Leveraging past translations for consistency.
- Human Post-Editing: Ensures the final output meets quality standards.
- Regular Evaluation: Continuously updating training data to improve models.
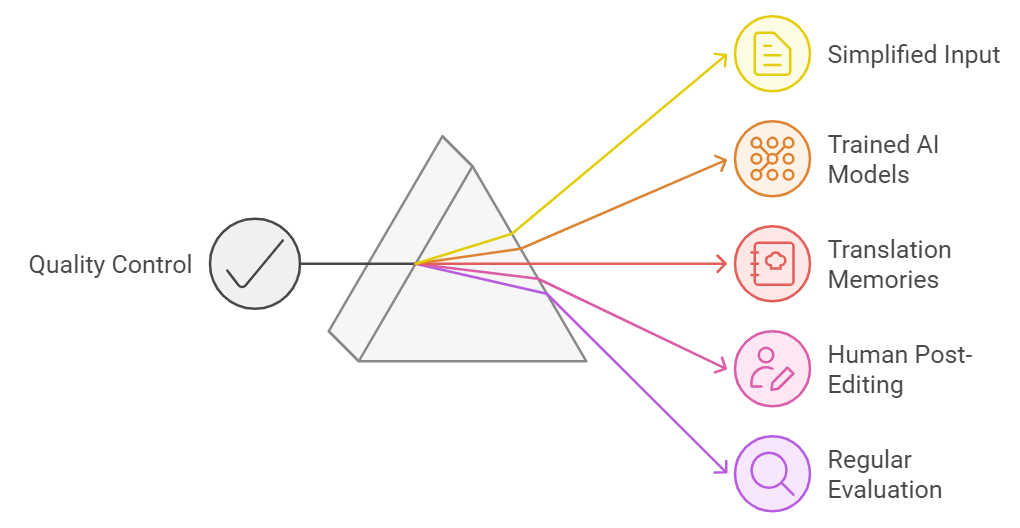
Ethical Considerations and Challenges
As with any technology, the integration of AI in various sectors comes with its own set of ethical concerns. These include data privacy, bias in AI models, and the potential displacement of jobs. Addressing these challenges requires careful data selection, transparent model training, and human oversight.
Ethical AI Deployment
- Data Privacy: Ensuring user data is protected.
- Bias Mitigation: Training models on diverse datasets.
- Human Oversight: Maintaining control to address unforeseen issues.
Future Trends and Developments
The future of AI and machine translation is incredibly promising. We can expect significant improvements in real-time capabilities, support for low-resource languages, and integration with other technologies like speech recognition and natural language understanding.
What to Look Forward To
- Real-Time Translation: Enhanced speed and accuracy.
- Low-Resource Languages: Better support for less common languages.
- Technological Integration: Combining MT with speech and NLP technologies.
Businesses are increasingly adopting AI to enhance global communication, streamline operations, and drive innovation. AI is becoming an indispensable tool in today’s interconnected world, offering endless opportunities for growth and development.
Latest Words
AI and machine translation technologies are revolutionizing various industries by improving efficiency, accuracy, and accessibility. While challenges remain, the continuous advancements in AI promise to further enhance global communication and operational efficiency, offering significant opportunities for businesses and individuals alike.
A Little Quiz for You!
- Who is considered the father of artificial intelligence?
- What is one major challenge still faced by neural machine translation?
- Name two platforms that provide AI-driven translation services.
- How is AI transforming the healthcare sector?
- What ethical consideration is crucial when deploying AI?
Feel free to share your answers in the comments below!